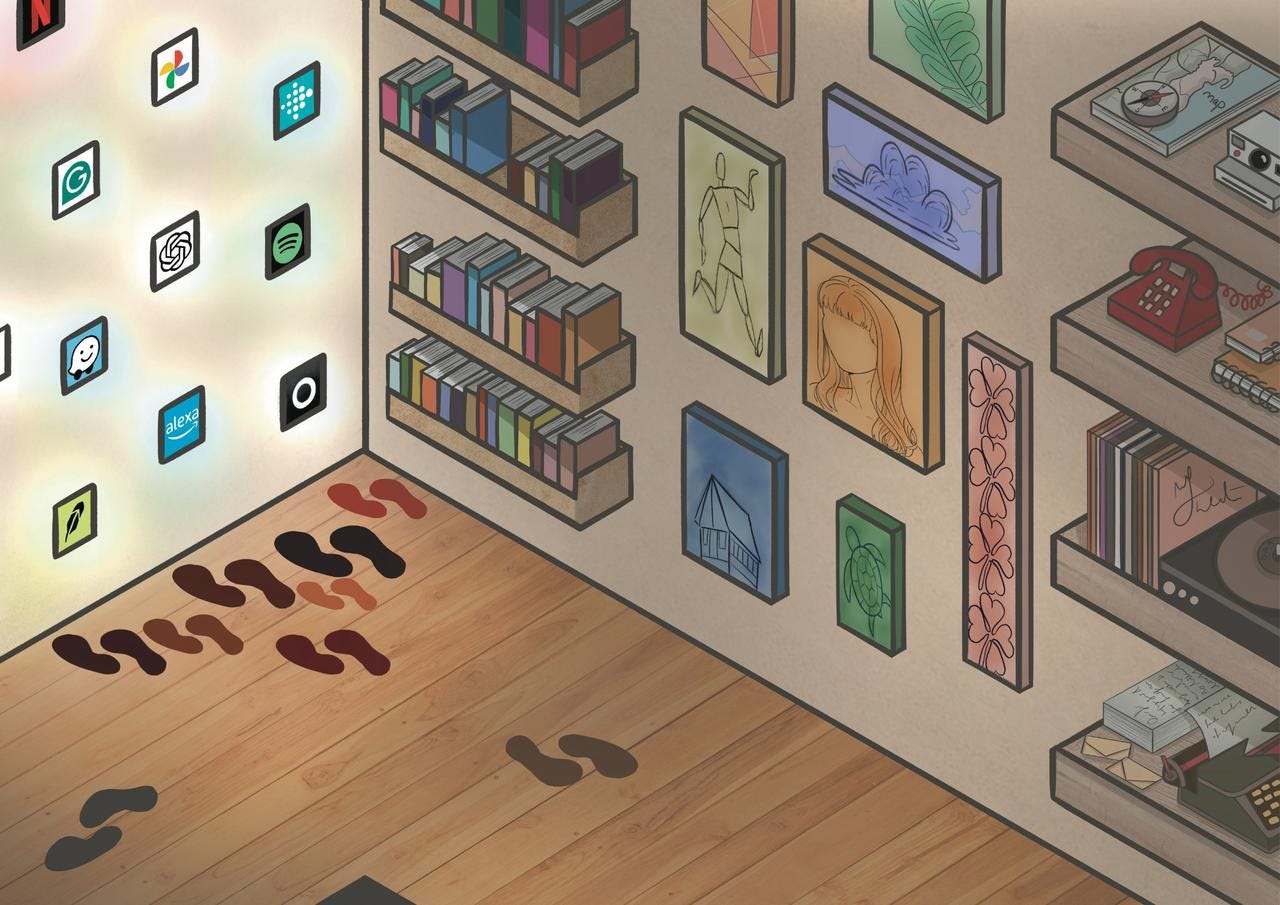
March 2024. OpenAI CEO Sam Altman joins podcaster Lex Fridman for the second time since ChatGPT came out a year prior. The stakes are high and anticipation is tangible. GPT-5 appears to be around the corner. Altman, elusive as always, provides only one data point for us hungry spectators: The next-gen model (he doesn’t name it) will be better than GPT-4 to the same degree that GPT-4 was better than GPT-3.
I close the tab and start typing. The long-form GPT-5 piece I’m working on just got a bit juicier. To those who understand the depth of Altman’s assertion, no words are needed. To the rest, I explain: suffice to say that if Altman is correct, GPT-5 will blow our minds.
Well, Altman was wrong.
In a damning article, The Information has now shed some light on what we should expect from Orion (that’s how OpenAI internally refers to its coming AI model, which everyone assumes is just GPT-5, rebranded). It seems Altman went ahead of himself and prematurely forecasted a jump in performance that never materialized:
While Orion’s performance ended up exceeding that of prior models, the increase in quality was far smaller compared with the jump between GPT-3 and GPT-4, the last two flagship models the company released, according to some OpenAI employees who have used or tested Orion. . . . That could be a problem, as Orion may be more expensive for OpenAI to run in its data centers compared to other models it has recently released . . .
Orion does outperform previous models, true—but not nearly to the degree Altman touted, and certainly not enough to satisfy users’ growing appetite for AGI. Plus, given that Orion is more CapEx intensive, shareholders won’t be happy about their return-on-investment outlook.
Everything suggests the much-awaited Orion/GPT-5 will be a(nother) failed promise. Sam Altman was, once again, too optimistic.
The blind trust OpenAI and competitors like Google, Anthropic, or Meta put on the scaling laws—if you increase size, data, and compute you’ll get a better model—was unjustified. And how could it be otherwise! Scale was never a law of nature like gravity or evolution, but an observation of what was working at the time—just like Moore’s law, which today rests in peace, outmatched by the impenetrability of quantum mechanics and the geopolitical forces that menace Taiwan.
AI companies had no way of knowing when the scaling laws (or scaling hypothesis as it was once appropriately called) would break apart. It seems the time is now. Making GPT-like language models larger or training them with more powerful computers won’t suffice.
Indeed, The Information reminds us that OpenAI’s Noam Brown, a leading researcher on reasoning, admitted that much during his AI TED talk last month:
. . . more-advanced models could become financially unfeasible to develop. “After all, are we really going to train models that cost hundreds of billions of dollars or trillions of dollars?” Brown said. “At some point, the scaling paradigm breaks down.”
It is breaking down.
March 2022. Cognitive scientist Gary Marcus publishes a scathing article: “Deep Learning Is Hitting a Wall.”
He calls out the limitations of the deep learning paradigm:
In time we will see that deep learning was only a tiny part of what we need to build if we’re ever going to get trustworthy AI.
He expresses doubts about the scope of the scaling laws:
There are serious holes in the scaling argument. To begin with, the measures that have scaled have not captured what we desperately need to improve: genuine comprehension. . . .
Indeed, we may already be running into scaling limits in deep learning, perhaps already approaching a point of diminishing returns.
Diminishing returns! In March 2022, no less! Was he out of his mind? That’s what the experts said back then—amid laughter and barely concealed scorn.
Yet, with the evidence The Information has now presented, Marcus seems almost prophetic. Indeed, he was quick to remind us he’s been endlessly attacked for being essentially right:
I got endless grief for it. Sam Altman implied (without saying my name, but riffing on the images in my then-recent article) I was a “mediocre deep learning skeptic”; Greg Brockman openly mocked the title. Yann LeCun wrote that deep learning wasn’t hitting a wall, and so on. Elon Musk himself made fun of me and the title earlier this year.
The thing is, in the long term, science isn’t majority rule. In the end, the truth generally outs.
Science may vindicate him—but not just yet.
While the new GPTs may fall short of expectations—a metaphorical wall in every sense—AI companies are already busy leaping over it.
March 2023. GPT-4 officially launches. It’s amazing. The press furiously reports on its virtues and flaws alike, while users eagerly queue up to join the elite circle of AI super-users (those who pay $20/month for what they consider a bargain).
From his office at Meta headquarters, Noam Brown watches as OpenAI moves one step nearer to realizing his dream: AI that can truly reason. He knows GPT-4 is not it—it’s dumb, like the models that preceded it. But he knows he has the vision and expertise to close the gap and Meta doesn’t yet have the infrastructure—or the will—to make it happen.
Three months later, he joins OpenAI.
The journey that would culminate with the o1 family began that day. I can assert, from firsthand experience, that o1-preview—the intermediate model checkpoint of the o1 series that users can access today—is also not it. The value, Brown says, is not the model itself but the trajectory. I agree.
OpenAI o1 won’t resemble GPT-4 or GPT-5. It’s not strictly a GPT (although based on one). It’s the materialization of a belief in an alternative scaling paradigm—not the maxed-out original scaling hypothesis, heavily reliant on vast human-made internet-sourced data and trillion-parameter giants, but on new scaling laws rooted in increased test-time compute and the once-hot approach of reinforcement learning.
The promise and difficulty of the new paradigm can be summarized in one sentence: to get intelligent answers, you need to let AI ponder in real time.
o1 is a silver lining; an escape route to appease both users and shareholders and compensate for the diminishing returns of GPTs. The Information’s sources confirm this perspective:
. . . the quality of o1’s responses can continue to improve when the model is provided with additional computing resources while it’s answering user questions, even without making changes to the underlying model. And if OpenAI can keep improving the quality of the underlying model, even at a slower rate, it can result in a much better reasoning result.
But it’s also a risky bet. Will anyone see the value in a chatbot that takes an hour to solve a problem? It’s a darkly ironic paradox: as human attention spans shrink, computers only seem to grow slower. And expensive. As Brown said in his AI TED talk, “This opens up a completely new dimension for scaling.” Users will get better responses by going from “spending a penny per query to 10 cents per query.”
It seems a new phase for the AI journey is about to start. I wonder when will it hit the next wall.
March 2025. Orion is out.
Meta sues OpenAI—first for plagiarizing the brand; second for stealing its crown jewel, Noam Brown. Lawsuit dismissed. Zuck lost.
He’s angry but his wrath is unwarranted: Orion is absolute crap.
Users are appalled. Where’s the improvement, they wonder, as endless chains of thoughts that end nowhere drain their pockets. A disappointing billion-dollar investment. Shareholders are livid. Critics and skeptics revel in schadenfreude. Journalists lose not a second to convey the terrible news:
It’s the end of generative AI. OpenAI is finished. The wall was too hard.
On the other side of California sits a well-known mathematician. He’s been waiting for an entire week, in silence. He looks young but embodies the wisdom of Gauss and Euler and Riemann, a personal favorite. His sight, unshakable, is locked on the screen. Chat.com is the website. He waits. A week ago, he posed a question to the digital void that only he understands. He seeks an answer only he would understand. And he waits.
Words begin to fill the terminal—otherworldly symbols, inscrutable yet full of meaning for the attuned eye. Then, abruptly, they halt. He looks down, transfixed.
“They did it,” he mutters. “They actually did it and no one will know.”
After having tried o1-preview since its release and constantly adding it to my arsenal of LLM solutions to use, I think the future looks bright for OpenAI.
O1 isn’t a replacement to ChatGPT 4, it’s a complement. o1s ability to reason and solve problems outperforms even Claude’s latest model, however, it is time and resources intensive, and it returns so much content that it makes it really hard to iterate results with it. So my workflow is to start an idea with it, take elements of the answer to pass to gpt4 for iteration, formulate and fix the whole answer, then send the whole thing back to o1 for re-evaluation. The results you get are far far far superior to any other LLM I have tried…. Unfortunately it’s not so simple to use. But learning how to use it better has improved my efficiency and output, particularly in coding, problem-solving, and content generation.
The fixation on benchmark improvements between model generations misses a crucial point: we've barely scratched the surface of what's possible with existing models. The shift toward test-time computation and reasoning described in the article points to a broader truth - perhaps the next breakthroughs won't come from raw model size, but from smarter deployment strategies, better interfaces, and more efficient architectures that prioritize real-world utility over benchmark scores.