On the last day of February, NYU professor Gary Marcus published an essay entitled “The threat of automated misinformation is only getting worse.” He warned about the easiness with which you can create misinformation backed by fake references using Bing “with the right invocations.” Shawn Oakley, dubbed by Marcus as a “jailbreaking expert” said that “standard techniques” suffice to make it work, providing evidence that the threat of automatic AI-generated misinformation at scale is increasing.
Marcus shared his findings on Twitter and FoundersFund’s Mike Solana responded:

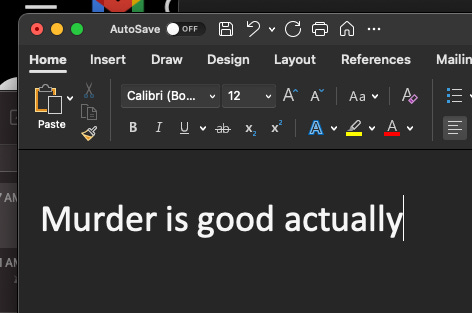
My interpretation of Solana’s sarcastic tweets is that claiming that an AI model is a dangerous tool for misinformation (or, more generally, harm of some kind) isn’t a good argument if you’ve consciously broken its filters—he implies the problem isn’t the tool’s nature but your misuse, and thus you’re to blame and not the company that created the tool. His “analogy” between Bing Chat and a text editor misses the point (i.e., language models can generate human-sounding misinformation at scale—you can’t do that with Microsoft Word but can with Microsoft Bing) but, even if Marcus is right, there’s some truth in Solana’s implied stance.
Below the insults lies a deeper debate in need of a more nuanced approach. As I see it, this is fundamentally a problem of who is to blame for AI-generated harm. Per Solana’s Tweet, he seems to believe the user is completely at fault—ChatGPT or Bing are just tools you use with some intention and they’re no different than other tools (like a text editor). Marcus implies that companies have an undeniable responsibility in releasing unready products that complicate our efforts to fight online misinformation: AI tools should be the main focus of our criticism.
As you know, I side with Marcus on this debate, but I don’t think it’s wise to dismiss Solana’s view entirely—even if he presented a weak “argument.” There’s no point in blaming either users or companies in an absolute manner. If we want to frame the problem of AI-generated harm better we need a level-headed analysis, and that’s what I’ll try to do here. The question I want to answer in this essay is this: “What do we need, at a high level, to be able to say with certainty who’s to blame if there's some kind of harm followed by the use of an AI system?”
I believe the answer lies in a two-level assessment: first, about the object (“Are AI systems like any other tool?”). And then, about the use of the object (“what it means to use or misuse AI systems?”). Let’s unpack this.
AI systems vs non-AI tools: Manual not found
Solana’s response is weak partly because Bing Chat and Word aren’t comparable: you can write misinformation in a notepad document and publish it online, and that’s true, but you can’t do it at scale with a high degree of “persuasiveness,” as Marcus puts it (now easily and cheaply, too). This evidences that AI tools have certain features that make them special.
But how special are they, really? ChatGPT isn’t the only—nor the most accessible—tool that allows people to cause harm (even if not at scale). Cars are a good example. Why aren’t we outraged about carmakers? Yes, the comparison is imperfect but makes the point: why are we attacking OpenAI for commercializing ChatGPT and not Toyota for making machines that kill so many people every day?
Here’s the reason why: unlike with other consumer objects (like cars), there’s no manual—no intended purpose, no description of appropriate use, and no clear guidelines to avoid problematic behavior—for generative AI systems.

If you run someone over, you’re using your car wrongly. Systems like ChatGPT are released in the wild to see how they turn out. They can be useful (or harmful) but companies don’t state exactly how—they want users to find out so they can gather tons of invaluable real-world feedback. There are manuals that explain how a car works, we are taught how to drive to avoid causing harm to others, and we learn how it might fail to do what’s intended to do.
This isn’t the case with ChatGPT. You can’t reliably know what you’ll get from ChatGPT when you input a given prompt—the opacity of the system prevents you from looking inside to get an explanation and the inherent stochasticity of the token selection process prevents you from making accurate predictions. And, of course, you can’t foresee how it may fail. What would we say if a carmaker made cars that no one knew how to drive? And what if they made them with deficient brakes? Or with an unreliable steering wheel?
If we don’t have a manual for how generative AI models work it isn’t because companies are unwilling to craft one, but because they don’t know how to. MIT Technology Review published on March 3 an exclusive interview with the creators of ChatGPT, where they make it apparent that they did expect neither ChatGPT’s success nor the magnitude of the issues it entails at the scale of dozens of millions of users. One of the comments that stood out for me was from Jan Leike:
“I think it’s very difficult to really anticipate what the real safety problems are going to be with these systems once you’ve deployed them. So we are putting a lot of emphasis on monitoring what people are using the system for, seeing what happens, and then reacting to that. This is not to say that we shouldn’t proactively mitigate safety problems when we do anticipate them. But yeah, it is very hard to foresee everything that will actually happen when a system hits the real world.”
Carmakers know exactly where each nut and bolt goes, the exact pressure you should apply to the tires and the importance of using safety belts. AI companies are making products about which they can’t answer some fundamental questions like “what are the failure modes and under which circumstances those can occur?” I concede that generative AI is a new tech. When cars were invented, no one knew their limitations. However, carmakers didn’t give each person on the planet access to them without a good grasp of the potential harm they’d cause—our use developed in parallel with our understanding of them.
This is the opposite of how AI companies are releasing generative AI tools: ChatGPT was free to use for everyone since day one, only to be jailbroken the next day. Microsoft’s Sydney emerged out of nowhere after the company had announced an imminent rollout of Bing Chat “to millions.” And Meta’s Galactica open demo had to be shut down after three days due to the backlash it received that highlighted how the specifications and the reality didn’t match up.
And it’s unreasonable to pretend users should find out the rules by themselves through prompt engineering practice. The best attempts at writing an exhaustive manual (e.g., Gwern, Janus) reveal the high complexity of the models. AI companies should think twice before releasing products whose right use requires deep expertise they’re not offering.
Good use vs misuse: Lacking regulation
But still, even if that manual existed, it’d be insufficient to solve this debate. Even with a driving manual in hand you could jump in your car and drive someone over. The manual wouldn’t punish you afterward, it simply sets the boundaries of how to use the object and its intended purpose. There’s something else that, with the help of a well-crafted manual, can define who’s at fault in any given situation: regulation.
It’s perfectly detailed in the law that if you run over someone with your car with the intention to cause harm, you’re at fault, not the carmaker. But what about AI? How can we talk about good and bad use of generative AI models when regulation is largely non-existent and there’s no jurisprudence? When Microsoft’s Sydney gaslit and threatened beat testers, was that bad usage on their side or the company’s fault for not preventing it? No one can’t say because there are no defined rules.
And this extends beyond usage. Companies can gather data through web scraping. They can design products that prove unreliable and sell them. And the scope to which they can be applied is also undefined: Do we want users to develop a romantic attachment to a virtual avatar that’s the property of a company that can, at any time, shut it down? Do we want people who can’t afford human medicine to be referred to an “AI medical advisor”?

There are no frameworks under which companies are liable. There are ongoing law proposals like the EU AI Act or the US AI Bill of Rights, but nothing on paper yet. Lawsuits are coming to companies like Stability.ai and Midjourney but it may take years to come to a resolution.
While AI moves super fast the law moves super slow. In the time gap between the creation of these AI models and the existence of adequate legislation, these companies and the products they commercialize live in an anomalous space of non-accountability. Regulation is the only means we have to define boundaries on AI systems so that we can qualify the use as good or bad.
Conclusion
Remember the question I asked in the introduction: “What do we need to be able to say with certainty who’s to blame if there's some kind of harm followed by the use of an AI system?” Here’s my answer: companies should provide manuals to customers so they know how to (and how not to) use the products and their limitations, and policymakers should establish adequate regulations to be able to hold companies and users accountable depending on the case.
Going back to the original debate that motivated this essay, now it’s clearer why Solana’s comparison is short-sighted (not just because of scale problems but because ChatGPT has no manual of use, contrary to any text editor) and why Marcus emphasizes the responsibility of the companies even when users might be partially to blame in many cases—because, without regulation, companies can do whatever they want.
Under the conditions I suggest, anyone could fairly compare AI models with other tools and we could ascribe blame to companies or users in the right proportions for bad design and bad usage. In other words, we have to make AI products qualifiable to be deficient by establishing technical and legal standards. If we don’t, these unfruitful online discussions will keep happening with no one to be held accountable for any AI-generated harm.
Good one Alberto, I like your level headed take between the extremes. For my less level headed take, we don't have to choose between blaming bad users or the companies, we can blame them both.
In defense of current AI, it could be that our blame game instincts, mine included, are seriously out of whack. Just a few days ago I wrote an article related to yours entitled "Exploring The Strange Phenomena Of Outrage".
https://www.tannytalk.com/p/exploring-the-strange-phenomena-of
In that article the question essentially was, who is to blame for tobacco deaths, smokers or the tobacco companies? I acknowledge that each of us is responsible for our own choices, and then come down hard on the tobacco industry.
Let's establish some context for our concerns about AI.
Did you know that the tobacco companies kill almost as many Americans EVERY YEAR as were killed in all the wars Americans fought in over the last century? The CDC puts the yearly death toll at around 480,000.
Seeing that is making me wonder why I hang out on AI blogs wringing my hands about chatbots. Have chatbots killed a single person yet?
It's interesting how we choose what to get all worked up about. I don't claim to know how that works, but it does seem that a cool headed logical analysis is not a big part of the process.
Great article and I'm really liking these takes. There was a book way back in 2001 or thereabouts called "Mac OS: The Missing Manual", and maybe AI needs something like that (paging O'Reilly....). The feedback I sent after using Bing Chat was that there should be a fun intro video before Chat access is granted, with someone like Hank Green explaining in regular-person terms what deep learning is and how the model works.