AI Emotion Recognition Is a Pseudoscientific Multi-Billion Dollar Industry
And it's us who are going to suffer the consequences.
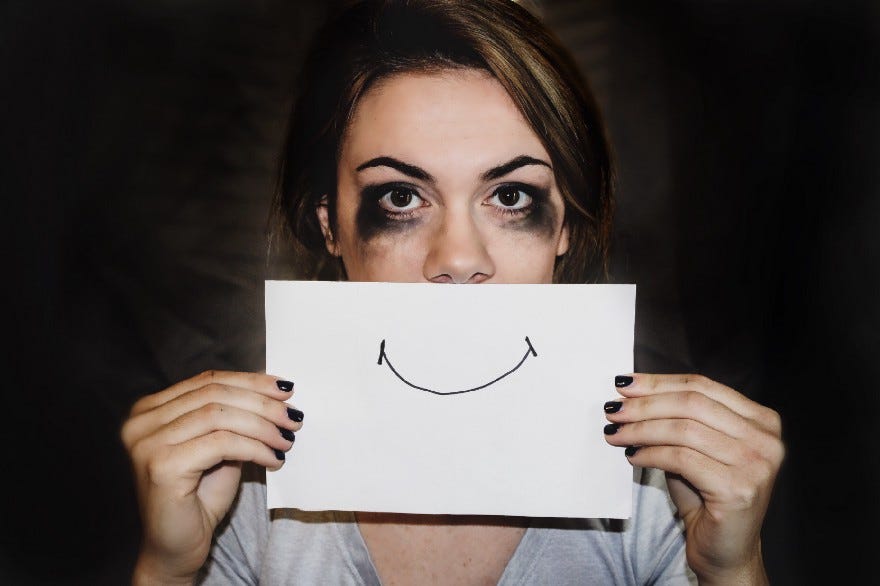
Tech companies are a key force for the progress of civilization. However, not even unlimited money and the best experts in the world can overcome the limits of science. It’s in those cases that the powerful CEOs should listen to scientists’ warnings and stop profitable endeavors to avoid harmful consequences.
AI-powered emotion recognition (ER) systems aim to detect and identify emotions in human faces. This controversial branch of AI has seen increased interest in the industry throughout the last decade (the market is expected to grow to $85 billion by 2025). However, ER doesn’t work as companies claim. Its lack of scientific grounding makes this otherwise attractive technology extremely problematic—especially for already discriminated minorities.
ER is part of a larger branch called facial recognition which is already embedded in services that we come across every day—most of them at the very least ethically questionable. It allows employers to evaluate potential employees by scoring them on empathy or emotional intelligence, among other traits. It helps teachers remotely monitor students’ engagement in school or while they do classwork at home. It’s used to identify “dangerous people.” And it has been implemented to control the US border with Mexico.
The tech giants, often early adopters of potentially lucrative technologies, soon understood the value of such systems and developed facial recognition software they now offer with their computer vision applications.
Those systems usually provide ER features that promise to predict emotions from facial gestures. But science says otherwise.
The unfounded promises of ER technology
2016 was the year of ER service announcements.
Microsoft released Face API, an algorithm they claimed could detect “anger, contempt, disgust, fear, happiness, neutral, sadness and surprise.” They further claimed that “these emotions are understood to be cross-culturally and universally communicated with particular facial expressions.”
Amazon launched Amazon Rekognition, a system that can supposedly identify basic emotions from faces in images or videos. And Google announced Google Cloud Vision API, which allows you to get “likelihood ratings for emotion (joy, sorrow, anger, surprise).” The company wanted to expand the system beyond those four emotions but its AI ethics team advised against it.
But not only well-established companies jumped onto the ER bandwagon. The drive to build these systems was so powerful that it gave birth to a significant number of startups.
HireVue, based in South Jordan, “compares candidates’ tone of voice […] and micro facial expressions with people who have previously been identified as high performers on the job,” write Hilke Schellmann and Jason Bellini for the WSJ. Eyeris is aiming at making cars “much, much safer than they are today” using facial and emotional recognition. Emotient, acquired by Apple in 2016, scans consumers’ faces in real-time to assess their emotional reactions to ads and products.
Affectiva, a company that emerged from MIT Media Lab in 2009, has been trying to tackle some issues related to the difficulty of extracting emotional states from facial information. Rana el Kaliouby, Affectiva’s CEO, says they want to incorporate “culturally specific benchmarks” to solve situations in which contextual and social factors influence the supposed universality of emotional expression.
But even Affectiva’s singularly careful approach may not be enough (and although they know this, they still partnered with HireVue). What’s the reason? ER technology doesn’t work the way all these companies claim.
ER technology is built on “shaky scientific ground”
The tech is based on Paul Ekman’s theory of basic emotions which states, in the words of Taylor Telford, that “six emotions—happiness, sadness, disgust, fear, anger, and surprise—are represented by universal facial expressions across all cultures.”
Ekman defended—and still does—that we can infer emotional states from facial expressions reliably. However, this notion has been challenged once and again and it’s now generally accepted that the evidence is inconclusive; emotion recognition technology is built “on shaky scientific ground.”
But let’s go back to see how this debate has been unfolding in recent years, what are the latest conclusions in the scientific community, and what it means for this multi-billion-dollar industry—and for us, the targets of ER services.
Paul Ekman, a psychologist at the University of California at San Francisco, based his approach to emotion research on the work of Princeton psychologist Silvan Tomkins. Tomkins thought that, as Kate Crawford puts it, “affects are an innate set of evolutionary responses.” But even he, the “forefather of affect research,” recognized that the specific displays of affect depended on “individual, social and cultural factors.” He acknowledged that ‘facial language’ was not spoken everywhere the same way.
Ekman wanted to formulate a universal theory that could overcome these issues. He thought that, if emotional expression was an evolutionary advantage, it should be universal across societies and cultures.
For the last 50 years, he has been improving his methods with the help of advances in computational power and the dramatic growth of available face datasets. In the first decade of the 21st century, his theories became widely accepted and his influence extended among scholars and the industry alike.
Yet, his methodology has been criticized regarding validity issues. Historian of science Ruth Leys argued that Ekman’s method was inherently flawed as he used data extracted in controlled environments, in which emotions were displayed artificially, ignoring individual and contextual variations. Leys claims that the face images he used were “already free of cultural influence.”
Happiness doesn’t always come from a smile—and not all smiles convey happiness
The most important criticism of Ekman’s work came from a review published in 2019 by psychologist Lisa Feldman Barrett and colleagues. The main purpose of the paper was to assess whether the scientific evidence was “sufficiently strong and clear enough” to justify the idea that we can infer emotional states from facial movements. They analyzed the literature on Ekman’s view—also called the common view—, by which an emotional state is unequivocally linked to a facial movement.
They evaluated the common view on emotion production (making facial movements when feeling a specific emotion) and emotion perception (inferring an emotion from facial movements) on three criteria: Reliability (for instance, whether happiness generates a smile most of the time), specificity (whether a smile is only generated by happiness), and generalizability (whether the happiness-smile relationship occurs also in real-world scenarios and across vastly distinct communities). They considered a wide range of populations: adults in the US, adults in remote rural communities, children, and congenitally blind people.
Barrett’s overall conclusion is clear: “It is not possible to confidently infer happiness from a smile, anger from a scowl, or sadness from a frown, as much of current technology tries to do when applying what are mistakenly believed to be the scientific facts.”
They found limited reliability (emotions don’t always generate the same facial movements), lack of specificity (emotion-facial movement pairs don’t present unique mappings), and limited generalizability (cultural and contextual factors haven’t been sufficiently documented).
Barrett argues that “perhaps unintentionally” the way scientists are conducting research is misguiding consumers of affect research — tech companies and other researchers — into thinking that there is a perfect mapping between emotion and face movements. From these conclusions, we can say two things: Ekman’s theory is at best partially wrong, and neither academia nor industry might be well aware of the degree to which it is wrong.
What’s the future of ER AI?
Barrett’s findings arose a new question: With this evidence, will the multi-million emotion recognition industry still commercialize products that don’t work as they proclaim?
To some degree, the new state-of-the-art in affect research has made the main actors in the industry rethink their approaches to emotion recognition.
HireVue announced in January last year they’d stop using visual analysis in their algorithms and expressed hope that “this decision becomes an industry standard.” Just a few weeks ago, Microsoft decided to stop providing open access to ER features on its Face API—except in some specific cases like helping people with vision loss. Many companies seem to have shifted their path to the one Affectiva was already paving some years ago.
However, although the latest research has shed some light on key issues of emotion recognition technologies, there’s one last question we should ask ourselves: Even if someday these technologies are perfectly unbiased, do we want governments and companies to have the power to understand our inner emotional states, anywhere, anytime?
Deborah Raji, who coauthored an important study revealing gender and racial bias in Amazon’s facial recognition system, said that even if the technology worked perfectly, it could be “easily weaponized against communities to harass them.”
The exposure of biases in face and emotion recognition technologies gave way to a more crucial debate. For now, the tech giants have stopped selling their products to governments, but regulation is yet to be implemented in the US. Even if the biases disappear, these systems could still jeopardize our privacy in ways we can only imagine.
Alexa Hagerty and Alexandra Albert say it best: “Technologies can be dangerous when they don’t work as they should. And they can also be dangerous when they work perfectly in an imperfect world.”
This is an updated version of an article previously published on Towards Data Science.
Imperfection is part of almost (if not all) current applications of AI. Should we stop using them because of this? How do you "scientifically" justified language generation advances in terms of any Science? Should we stop because it generates gibberish?
"Caminante no hay camino, se hace camino al andar" (Antonio Machado Ruiz, poet)